This article by Matt Danford originally appeared in Modern Machine Shop magazine.
I’ve written before about how supplementing CNC data feeds with additional information can evolve machine monitoring beyond surface-level uptime and downtime analyses. With real-time conditional data on spindles or cutting tools, the system also becomes a platform for predictive maintenance, in which the changing of end mills and the greasing of spindle bearings is governed by actual need rather than a regular (and perhaps overly conservative) schedule. But what if such capability could be obtained without supplementing the data feed — that is, without installing new sensors?
MachineMetrics, the provider of a cloud-based data platform for collecting and analyzing shopfloor data, has been working for a few years to obtain the necessary insights from the equipment’s native sensors alone. Based on early applications, this approach can be just as effective and easier to scale.
What’s more, ambitions extend beyond condition monitoring of cutting tools and spindles. The primary goal of enriching CNC data feeds is not to add capability to the machine monitoring system, says Bill Bither, CEO of MachineMetrics. Rather, it is to provide a platform for solving a variety of user-specific problems with a variety of user-specific analysis methods. As he explains it, “We provide access to contextualized machine data and no-code workflows that enable users to solve all sorts of use cases with machine data that we could never have imagined.”
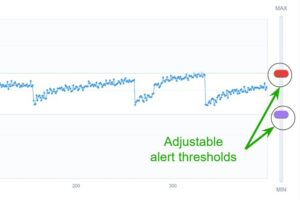
The CNC data feed is “enriched” in the sense that it is high frequency; that is, the points are collected at a rate of one thousand points per second (1 kHz), compared to a typical rate of only one hundred (1 Hz). Although the latter, slower rate is more than sufficient for determining whether machines are running, flagging predictive events in near-real-time leaves no room for wide gaps between data points. Likewise for stopping a machine, correcting an offset or otherwise correcting any anomaly the monitoring might detect.
High-frequency data is also critical for extrapolating information that cannot be measured directly from the CNC. For monitoring tool condition, most users seek predictive signals in spindle power readings, which increase as cutting edges deteriorate. Depending on the application, a user might also wish to zero in on acceleration, friction or any other value that might be derived from spindle power data. In some cases, notifications or corrections can be issued when torque, spindle speed or any other measurable metric reaches a certain threshold.
For now, the focus of high-frequency data collection is FANUC CNCs, although work continues with others. Whatever the control type, merely enriching the data feed is not enough. The more granular the data, the greater the level of “noise” — meaningless information that must be filtered out before true insight can emerge. For example, fluctuating lathe spindle power might be due to cutting effects that indicate tool wear, or it could be a result of a barfeeder changeover. Normalizing the data —– that is, labeling, defining and otherwise formatting it for analysis —– was also a prerequisite for making machine monitoring a platform for condition monitoring, whether with the company’s own algorithms or those that have yet to be developed.
Regardless, Bither says the essentials are in place for users to focus almost entirely on application rather than setup. Although MachineMetrics is cloud-based, condition monitoring is largely a localized endeavor. The processing for high-frequency CNC data collection and normalization occurs on the edge device itself: a box-like industrial computer at each machine that is the system’s only hardware component.
However, external sensors are still supported for applications that might benefit from data beyond the equipment’s native sensors (for example, analysis that involves tracking coolant consumption and costs). In fact, the company recently partnered with IFM, a provider of sensors that use IO-Link technology (a wireless communications protocol that works similarly to Bluetooth).
If possible, however, eschewing sensors entirely is likely to be the more scalable approach, Bither says. Third-party sensors are subject to bumping or other physical interference, and their output also can be clouded by dirt, chips, coolant, extreme temperatures or various other hazards of harsh, industrial environments. Where to place and how to power the sensor are also concerns. “We’ve had to do an awful lot to simplify the whole process of getting the data and the tools to analyze it and build workflows around it,” he concludes. “That simplification makes (condition monitoring) possible.”